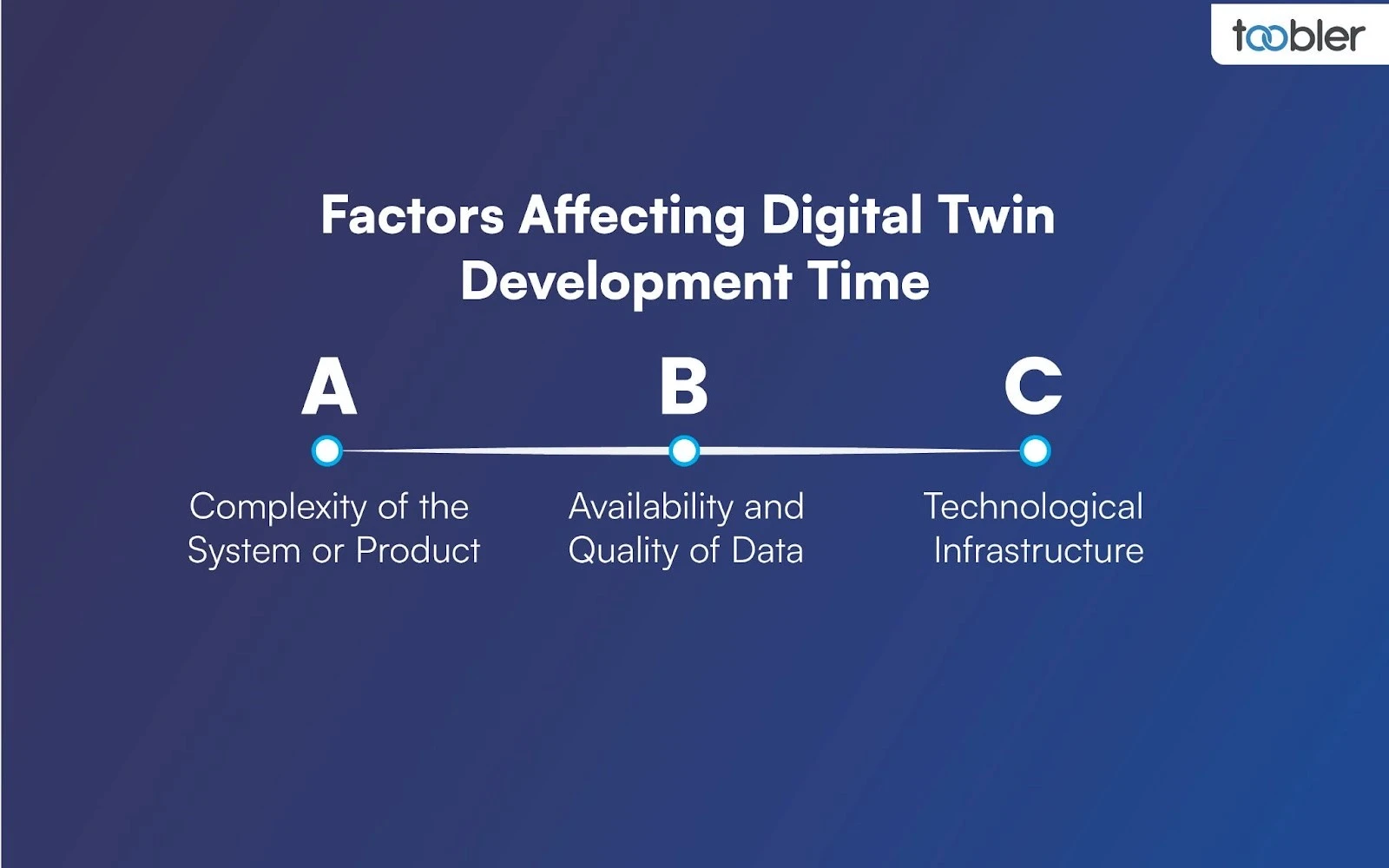
1. Complexity of the System or Product
The primary determinant of digital twin development time is the complexity of the system or product under consideration. More sophisticated connections and many elements in a system call for more thorough simulation and modeling. For instance, consider digital twins in manufacturing. Creating a digital twin for an essential piece of machinery can take a few months. Conversely, creating a digital twin for a whole manufacturing facility or significant infrastructure project might take many months or even years.
The time needed is increased by the complexities of precisely reproducing each part and procedure, guaranteeing the model's fidelity, and connecting different subsystems. A more thorough and precise digital twin involves a more prolonged development procedure. In contrast to an essential mechanical device, the digital twin of an aviation engine requires modeling multiple components. This entails each with specific behaviors and relationships, which results in lengthier development durations.
2. Availability and Quality of Data
The growth of digital twins depends on data. The quantity and quality of data significantly influence how long it takes to generate a functional digital twin. High-quality, real-time data from sensors and Internet of Things devices precisely mirrors the activity of the digital twin of a physical asset.
Conversely, inconsistent or low-quality data can result in erroneous models and require more effort for data cleansing and testing.
Gathering data from multiple sources, such as previous databases, real-time sensor feeds, and manual inputs, and ensuring that it is integrated and synchronized can take a while. Installing sensors, setting up data collection methods, and guaranteeing data integrity could also take more time if the required data is not easily accessible.
For example, information from occupancy sensors, lighting, HVAC systems, and other sources is needed to create digital twins for smart city development. Optimizing the availability and accuracy of all these data pieces can significantly influence the timeframe.
3. Technological Infrastructure
The time it takes to construct a digital twin also depends mainly on the available technology infrastructure. The development process can be streamlined with sophisticated simulation tools, powerful data processing skills, and easy integration networks. Data integration, simulation, and gathering can be accelerated if the required technology infrastructure is currently in place.
Conversely, insufficient or antiquated infrastructure might hinder development efforts by making upgrading systems, finding new tools, and guaranteeing compatibility more challenging.
For example, a company that relies on outdated technologies that require significant changes cannot generate digital twins as quickly as one that uses a current IoT platform and sophisticated simulation tools.
Furthermore, the development team's proficiency and familiarity with the selected technology may impact the timeline. A knowledgeable team with up-to-date knowledge of digital twin technology can overcome obstacles faster and finish projects sooner. This is where the best digital twin companies play a pivotal role.
Please Read: How Toobler Helps Companies Become Digital Twin Ready? Here
