
A virtual model created to replicate a real system, item, or procedure accurately is called a "digital twin." By utilizing digital twin technology, companies can establish a connection between the virtual and real worlds.
This technology enables continual tracking and simulation of real-world assets, allowing for the prediction of possible outcomes. Consequently, it facilitates analysis without the need for direct interaction with the physical world.
It functions basically as a lively, real-time reflection of a physical object, optimizing its efficiency and making choices using analytics and data.
Key Components of Digital Twinning and How They Work
To fully realize the potential of digital twinning, a few essential elements must cooperate:
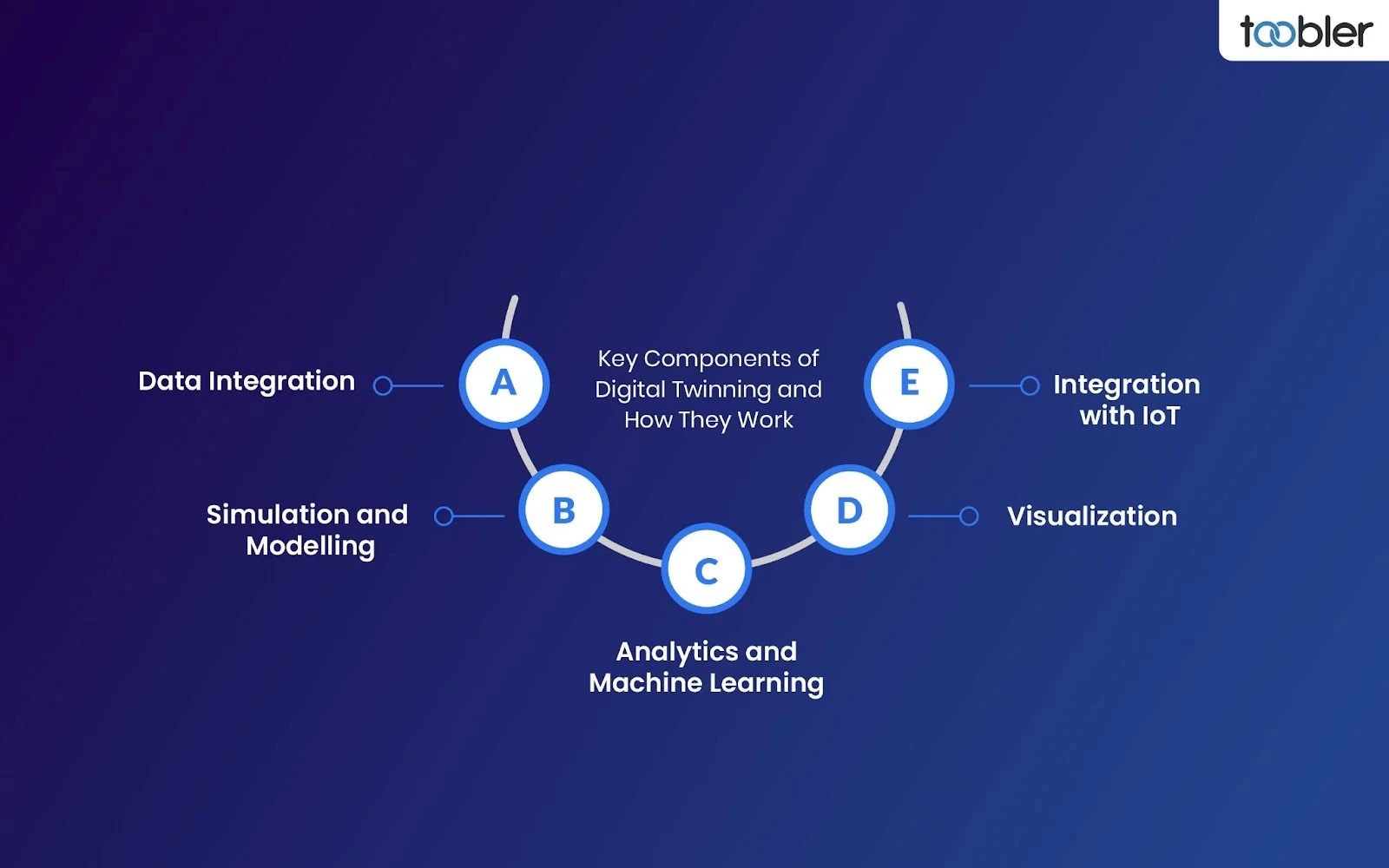
1. Data Integration
A digital twin's core involves integrating data from multiple sources, such as operational, environmental, and sensor data from physical assets. This data is constantly gathered and injected into the digital twin to maintain the model's accuracy and currency.
Data integration is widely used in the aerospace sector, for instance, in the digital twins of aircraft engines. During a flight, these digital twins collect sensor data, atmospheric information (such as humidity and air density), and operational info (such as temperature and pressure) straight from the aircraft's engines.
2. Simulation and Modelling
Digital twins can use sophisticated simulation tools to simulate the behavior of a physical asset under different conditions. These simulations are essential for testing scenarios in a safe environment so that optimization can take place prior to any real-world implementation.
Imagine the automotive sector, specifically with regard to the creation of electric cars (EVs). To replicate how EV batteries will behave in different driving scenarios and climates, digital twins of the batteries have been developed. This simulation shows how battery lifespan, discharge rates, and performance degrading are impacted by temperature variations. Automotive engineers may improve the battery management systems without directly manufacturing and testing every variant by employing these models.
3. Analytics and Machine Learning
Through analysis of the collected data, designs, results, and suggestions for improvement are identified. As a result of machine learning algorithms' ability to learn from the data over time, the digital twin technology becomes wiser and more effective at anticipating problems in the future or streamlining processes.
For example, machine learning methods are utilized by digital twins of wind turbines to examine data obtained from multiple sensors on the turbines. Temperature, torque measurements, wind speed, and turbine speed are all included in this data. From this data, machine learning algorithms can learn to maximize energy output, forecast component failures, and recommend maintenance plans. This predictive ability makes sure the turbines run as efficiently as possible, extending their lifespan and raising the wind farm's total productivity.
4. Visualization
Decision-makers can more easily comprehend complex data and simulations because of the advanced visualization features that digital twins frequently offer. This might include everything from straightforward 2D schematics to intricate 3D models and augmented reality (AR) settings.
For example, engineers and architects can visually walk through the digital twin of a building on-site using AR overlays. This helps them to see prospective structural alterations or systems within their real-world context in construction and real estate. This ability to visualize how the modifications might look and behave in the real world significantly improves comprehension and decision-making.
5. Integration with IoT
The IoT and digital twins work together. Real-time data is collected from the physical asset by sensors and IoT devices, which then relay it back to the digital counterpart for instant analysis.
Imagine an assembly line in a manufacturing plant that uses digital twins. IoT sensors that are mounted on different pieces of equipment gather information on operating parameters including temperature, speed, vibration intensity, and energy use. This information is fed into the digital twin, an exact replica of the real production line.
Real-time data analysis is done by the digital twin to find any anomalies or inefficiencies. The digital twin can notify the maintenance team right away if a machine starts to operate outside of its ideal temperature range so that the problem can be fixed before it causes a breakdown. This preventive measure contributes to reducing downtime and preserving steady production flow.