Data Integration and Management
One of the most difficult aspects of using digital twins is integrating several data sources. To remain accurate, these virtual duplicates depend on an ongoing flow of data from sensors, IoT devices, and enterprise systems.
However, data silos—where vital information is segregated within departments or platforms—are a problem for many businesses. This makes it more challenging to create a seamless digital twin.
To solve this, a strong data architecture that can manage real-time processing and data synchronization is needed. Data must also be cleansed and checked regularly to ensure the digital twin truly matches its real-world counterpart. Without a robust integration and management plan, the digital twin's efficacy may be affected.
Also read: The Benefits and Challenges of Digital Twins in Construction
Security and Privacy Concerns
Cybersecurity is a significant worry because digital twins store enormous volumes of information about processes and services. Unauthorized access may reveal private information, such as real-time performance insights or product specs, which could reduce a company's competitive advantage.
Personal information, such as user or employee interactions, is frequently included in digital twins and is subject to data privacy regulations like GDPR. Organizations require multi-layered security solutions that include encryption, access restrictions, and frequent security assessments to safeguard this data.
Setting security measures as a top priority ensures data integrity across the product lifecycle, protects private information, and fosters stakeholder trust.
Further read: Digital Twin Security: Steps to Take & Best Practices
High Implementation Costs
High upfront expenses are associated with the implementation of digital twins, encompassing everything from data infrastructure to qualified staff. After initial setup, finances may be strained by the requirement for continuous maintenance, updates, and staff training, especially for smaller businesses.
Although encouraging, the payback period can be lengthy as businesses progressively get access to predictive maintenance tools and lower prototyping costs. Companies worried about their budget may find that a small-scale pilot initiative works well.
This enables companies to verify the advantages of digital twins before extensively implementing them, maximizing operational and financial assets.
Also read: Overcoming Digital Twin Implementation Hurdles | Toobler
Future Trends in Digital Twin Technology
Exciting developments are emerging as digital twin technology develops further, which could increase the tools' strength and industry-wide adaptability.
Let us examine potential future paths, such as how digital twins develop alongside AI, enter new markets, and facilitate real-time data processing.
Suggested read: What is the future of digital twins?
Integration with AI and Machine Learning
Digital twins will advance to new heights with the help of AI and machine learning. AI will make digital twins more self-aware and predictive, enabling them to spot trends in data and make intelligent corrections without human assistance.
Consider a digital twin for an energy system that can modify power distribution in response to current consumption trends. Or else, consider a digital twin for a manufacturing facility that can anticipate equipment breakdowns by learning from historical patterns.
Because of these improvements, digital twins will actively optimize and upgrade physical systems in addition to mirroring them. With the integration of AI, digital twins are changing from static models to intelligent collaborators in product creation.
Suggested read: How is AI Enhancing Digital Twin Capabilities?
Expansion into New Industries
Although the manufacturing, healthcare, and automotive industries have long used digital twins, other businesses that want to use data to gain a competitive edge are also taking notice.
Digital twins in agriculture, for example, might be used to forecast yields, monitor soil health, and optimize crop management. Similarly, store managers in the retail industry can use digital twins to estimate demand, monitor stock levels, and even customize client experiences.
Digital twins provide a flexible way to track, forecast, and improve operations in new areas as sectors adopt data-driven strategies more and more, creating opportunities for innovation in unanticipated domains.
Advancements in Real-Time Data Processing
Digital twin technology is moving toward real-time analytics and decision-making in response to the need for real-time data processing. As communication and processing power increase, digital twins can react to conditions quickly rather than just monitoring them.
A fleet digital twin, for instance, might continually optimize delivery routes in transportation logistics by taking into account real-time traffic data, weather, and vehicle status. This helps give dispatchers and drivers real-time updates.
Because of this change to real-time responsiveness, digital twins can be used as proactive instruments for prompt decision-making. This enables companies to take action on insights as they arise rather than after the fact.
Please read: Importance of Real-Time Integration in Digital Twin
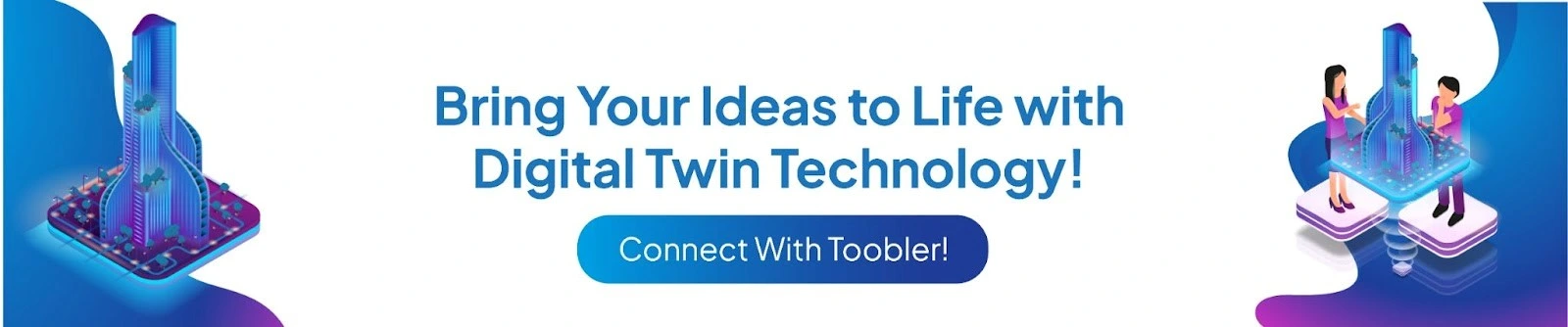