Technical Challenges
1. Data accuracy and quality
Since the digital twin is created using Model-based Systems Engineering(MBSE) and sensor fusion from various data sources, data fidelity is crucial. A digital twin with inaccurate or insufficient data may not accurately represent the vehicle, affecting its operational and maintenance activities.
For example, accurate data on the performance of individual components, such as fuel injectors or bearings, is critical for simulating and optimizing the entire system's performance.
However, collecting data for creating a digital twin ecosystem is also challenging - you may have to gather data from multiple sources and integrate them properly. Collecting and processing data can also raise privacy, security, and storage concerns.
2. Integration and interoperability
Digital twin ecosystems are complex systems featuring different types of data obtained from sensors, IoT devices, databases, BIM models, and many more. Therefore, automotive experts must consider data exchange protocols to ensure a smooth data transfer between the systems.
In addition, since different sources use different types of data, the digital twin must be designed to interpret various data types. Security is another factor that must be considered while creating a digital twin using various data sources.
Also Read: Importance of Real-Time Integration in Digital Twin
3. Scalability
When it comes to scalability, one of the main challenges is the sheer volume of data that needs to be processed. As more sensors and data sources are added to the system being modeled, the volume of data can quickly become overwhelming. This can lead to challenges in storing, processing, and analyzing the data in real time.
Complexity is another challenge faced while scaling Digital Twins. For example, a vehicle could have numerous amount of components that need to be accurately modeled and simulated if you want to create a reliable Digital Twin. For this, it might require significant computational resources, which can be difficult to scale up. Why? Because the system being modeled becomes more complex.
So, when designing digital twin solutions for complex automotive systems, you should have scalability in mind.
Please read: The Evolution of Digital Twin Software in Different Industries
4. Real-time simulation
Computational complexity is one of the main limitations in real-time simulations. Since creating a Digital Twin model for vehicles requires a large number of calculations, the process can be computationally intense. This requires high-performing computing resources, which is expensive and may limit scalability.
Also, it should be noted that for real-time simulations to perform well, they need accurate data inputs. But real-world data may not capture all relevant factors that could affect the accuracy of the Digital Twin.
Finally, the real-time simulation might not be suitable for testing certain scenarios or conditions. For example, conditions like extreme weather or rare system failures are hard to replicate in real life. This poses a challenge in testing the reliability of digital twins in such conditions.
Also read: Digital Twin Security: Steps to Take & Best Practices. Here
Security and Privacy Concerns
1. Data security & privacy
As mentioned earlier, the digital twin ecosystem operates on data obtained from various sources, including sensors, IoT trackers, and BIM models. It may also include sensitive data such as trade secrets, personal details of customers, proprietary data, etc.
So it is important to keep all this data safe from falling into the wrong hands. Unauthorized access can affect the reputation of the company and business operations, sometimes even leading to financial losses.
Companies must follow strong security measures like encryption, access controls, and firewalls to avoid data breaches and misuse. In addition, they can use secure storage solutions to safeguard the data. Regularly testing the system for vulnerabilities and security gaps is another way to ensure data security.
Legal and Regulatory Issues
1. Compliance
Protection of data is crucial, as was covered in the section before this one. Therefore, in order to preserve client privacy, adherence to GDPR, CCPA, or HIPAA standards is crucial.
The application of cutting-edge technologies presents a barrier in adhering to these laws. For example, techs like machine learning are used in optimizing the manufacturing process. But it can be difficult to prove whether these algorithms comply with relevant regulations and standards.
Thus, it is crucial to establish clear guidelines and standards for using different types of digital twins. This may involve working with regulatory bodies and standard organizations.
2. Intellectual property
Intellect Property, or IP, is valuable for companies that create and use Digital Twins. It can include patents, trademarks, copyrights, and other forms of proprietary information.
But, concerns related to the ownership and protection of IP in Digital Twin solutions need to be addressed. This is mainly because of the involvement of collaboration between multiple companies.
To give you a clear idea, consider the following example. Two companies collaborate to create a digital twin, a manufacturer, and a software company.
One company has exclusive data on the design and function of a specific component, while another has exclusive algorithms for simulating and improving its performance. If proper regulations and agreements are not in place, there is a risk of the latter's intellectual property being used without permission.
That's why clear agreements and contracts must be established to establish ownership and usage rights to protect data.
Organizational Challenges
1. Adoption barriers
While Digital Twins can revolutionize organizations' performance, there exists an adoption barrier. These barriers can include fear of change and lack of understanding.
Digital Twins are disruptive and can significantly change existing workflows and practices. However, some individuals or organizations may resist due to uncertainty about its impact on their job or the organization as a whole.
Digital Twin technology can be complex, requiring specialized knowledge and expertise. And individuals or organizations that lack this expertise may be hesitant to adopt it. Many people don't use it or prefer it because they don't fully understand its advantages and how to use it properly.
Organizations may also be hesitant to adopt this considering the complexity and cost of data management.
2. Skillset requirements
It's not always easier to find laborers with the right skill set to develop, implement, and maintain digital twins. Software development, data analytics, and simulation are a few of the areas laborers need to be skilled in.
Furthermore, it should also be noted that the Digital Twin tech is changing with the emergence of new technologies and techniques.
So, how to address these challenges?
The best and most affordable way will be to partner with a digital twin development company.
The best and most affordable way will be to partner with a digital twin development company.
Why?
Because they have more experience and expertise in developing Digital twins. They will have proper team and project management processes set in place, guaranteeing timely delivery of the product. And most importantly, they can help you improve digital twin with their expertise.
In regard to the budget, it will be cheaper than hiring an in-house team.
3. Cost considerations
Adopting Digital Twin technology can require a significant financial investment. This is because it involves acquiring specialized hardware, software, and expertise.
Also, the cost varies depending on the scope and complexity of the project. For example, developing a Digital Twin for a single component or subsystem of a vehicle may require less investment. At the same time, developing a Digital Twin for an entire vehicle or manufacturing process.
Despite these investments, the digital twin can contribute to several potential returns on investment. For example, the digital twin can help automotive engineers to detect potential machine failures and perform timely maintenance and services. In doing so, they can reduce machine downtime and improve its life. The digital twin can also be used to simulate real-world conditions and deliver personalized solutions, improving sales and ROI.
Meanwhile, for a successful digital twin implementation, you must connect with the best digital twin companies. This will help you remove the major challenges and limitations mentioned above.
Please read: How much does it cost to develop a digital twin
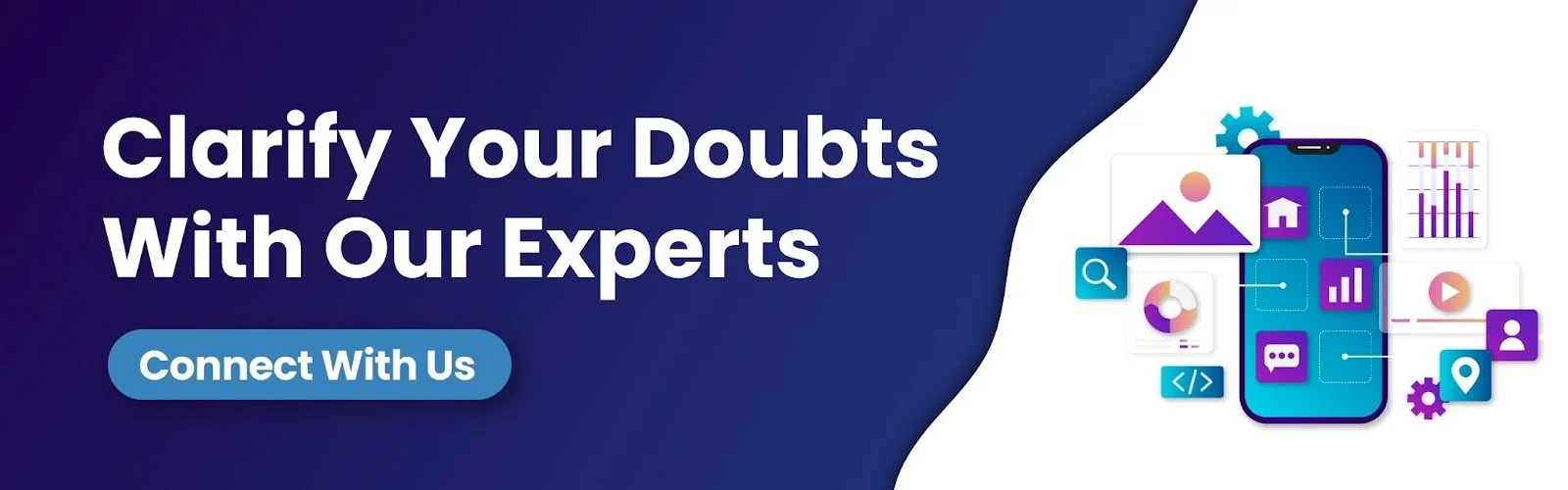